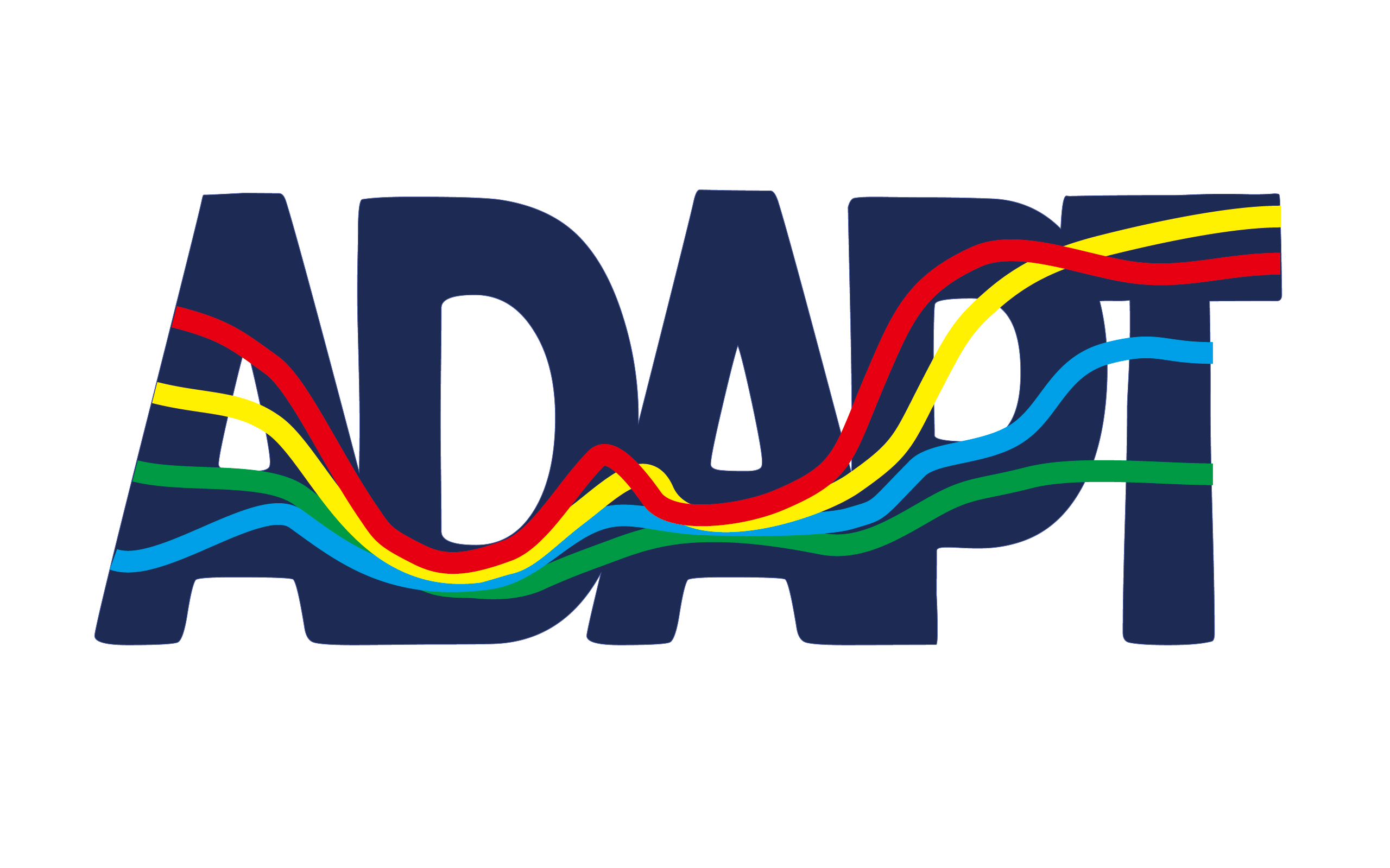
Mission Statement
The Penn State Center for Advanced Data Assimilation and Predictability Techniques (ADAPT) seeks to integrate and enhance the existing strength and expertise in cutting-edge data assimilation (DA) and predictability research across Penn State which span over several academic colleges (Earth and Mineral Sciences or EMS, Science, Engineering, Agriculture, and Information Science and Technology or IST) and research institutes (ICS, PSIEE and EESI). The primary objectives and activities are:
1) Design and develop advanced and efficient DA and predictability algorithms for automatic and accurate estimation of the state and uncertainty of dynamical systems building on both existing ensemble-based and variational methods.
2) Implement these advanced methods with fast efficient numerical solvers and parallel computing capability that will be suitable for a wide range of applications and in particular for numerical weather, water, air quality, climate and ecosystem prediction.
3) Apply the advanced DA and uncertainty qualification methods to assess the observability and predictability of various dynamical systems of interest, to improve the accuracy and design of various forecast systems, to assess the effectiveness and impact of existing observing networks, to design the most cost-effective future observing systems through observing system simulation experiments and/or pilot real-time real-data predictions of weather, climate and environmental hazards.
4) Serve as a natural home for facilitating interdisciplinary research across the Penn State campus, as a hub for attracting national and international students and scholars, and as a testbed for transferring research to operations. Be uniquely positioned as the first research center hosted by a US university in such a key research area, and be well aligned with the recent NSF initiatives on Cyberinfrastructure, BigData and EarthCube. Serve as a launching pad for applying for a federally sponsored research institute on advanced data assimilation and predictability techniques at Penn State.
Background
Data assimilation (DA) is the process of finding the best estimate of the state and associated uncertainty by combining all available information including model forecasts and observations and their respective uncertainties. DA is best known for producing accurate initial conditions for numerical weather prediction (NWP) models, but has been recently adopted for state and parameter estimation for a wide range of dynamical systems across many disciplines such as ocean, land, water, air quality, climate, ecosystem and astrophysics. Taking advantages of improved observing networks, better forecast models and high performing computing, there are two leading types of advanced approaches, namely variational data assimilation through minimization of a cost function, or ensemble-based data assimilation through a Kalman filter. Both DA techniques seek the best-linear unbiased estimate (BLUE), though more recent studies have demonstrated the impact of non-Gaussianity and nonlinearity assumptions. These two prevailing DA methods are mathematically equivalent under linear Gaussian system but can be drastically different in implementation and performance for complex nonlinear systems like the weather and climate. Each has its own advanced advantages and limitations. There is a growing trend in hybridization and coupling of these two classes of methods that combines strengths from component DA methods. For dynamical systems with smaller state dimensions, there is also a growing trend of exploring even more advanced but computationally more expensive approaches such as particle filtering.
Predictability is the ability to predict accurately the future state of a dynamic system and related uncertainties given inherent uncertainties in the initial conditions and/or forecast models that represent the dynamical systems. Broadly speaking, there are two fundamentally different definitions of predictability. Intrinsic predictability can be defined as 'the extent to which prediction is possible if an optimum procedure is used' in the presence of infinitesimally small initial errors. Practical predictability, on the other hand, can be specified as the ability to predict based on the procedures that are currently available. Practical predictability is limited by realistic uncertainties in both the initial states and the forecast models, which in general are not infinitesimally small. Understanding of the limit of predictability and the associated error growth dynamics is essential for setting up expectations and priorities for advancing deterministic forecasting and for providing guidance on the design, implementation and application of ensemble prediction systems. Over the past couple of decades, ensemble forecasting (EF) that incorporates the uncertainties in either the initial conditions and/or forecast models due to both practical and intrinsic limit of predictability has emerged as a powerful tool for uncertainty quantification and probabilistic prediction for numerical weather and climate prediction.
Following the success of NWP models, both DA and EF are now widely used for many other dynamical systems across various disciplines of science and engineering that include but are not limited to: astrophysics, planetary atmospheres, ocean modeling, hydrology, petroleum engineering, solid earth, earthquakes, storm surge, forest fire, land surface and land cover, air/water quality, and more broadly climate, ecosystem, and earth system modeling. DA also evolves from not only seeking the best estimate of the initial conditions, but also to understanding the error/uncertainties in the forecast models (often known as parameter estimation or PE) that represent the system dynamics. In the meantime, the complexity and development of DA and EF techniques for high-dimensional, non-linear dynamical systems have drawn interests from increasingly large number of applied mathematicians, statisticians, electrical/industrial engineers, and computer scientists.
Projects
- ADAPT - A BigData and Cyberscience Center
- Fuqing Zhang - PSU EnKF Realtime Atlantic Hurricane Forecast
- John Harlim - Nonparametric Modeling and Stochastic Parameterization
- Steven Greybush - Lake Effect Snow, Mars Atmosphere and Aerosol Reanalysis
- Le Bao - Bayesian Methods and Computational Methods
- Alfonso Mejia - Flood Forecasting
- Guido Cervone - Big Data Analysis, Hazards and Renewable Energy
- Jose Fuentes - Regional Transport and Chemistry Models
- Jia Li - Statistical Learning, Data Mining and Image Analysis
- Qunhua Li - Statistical modeling for complex data, clustering and classification
- Xiaofeng Liu - Environmental Fluid Dynamics and Computational Modeling
- Paul Markowski - Convective Storms and their Attendant Hazards
- Yvette Richardson - Severe Storms
- David Stauffer - High Resolution Prediction / Data Assimilation Systems
- James Wang - Cloud Motion Estimation and Classification Using Big Data
- Xiaolong (Luke) Zhang - Information Visualization and Mobile Computing
- Bu Zhong - Weather Impact on Reporting the Olympics, Information flow empowers vegetable farmers